When you study an abstract subject like linear algebra, you may wonder: why do you need all these vectors and matrices? How are you going to apply all this inversions, transpositions, eigenvector and eigenvalues for practical purposes?
Well, if you study linear algebra with the purpose of doing machine learning, this is the answer for you.
In brief, you can use linear algebra for machine learning on 3 different levels:
- application of a model to data;
- training the model;
- understanding how it works or why it does not work.
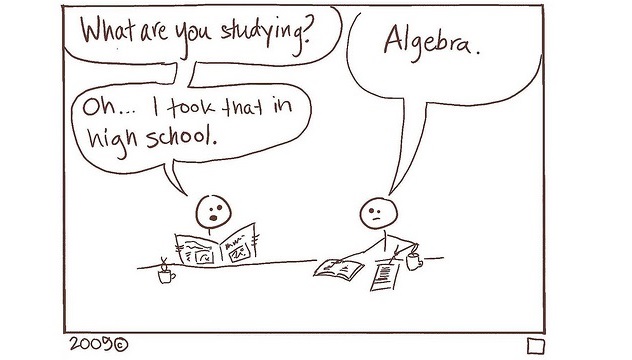
I assume that you, the reader, have at least a vague idea of linear algebra concepts (such as vectors, matrices, their products, inverse matrices, eigenvectors and eigenvalues), and machine learning problems (such as regression, classification and dimensionality reduction). If not, maybe now it's a good time to read about them in Wikipedia, or even signup for a MOOC on these subjects.
Application
What machine learning usually does is fitting some function , where is the input data, is some useful representation of this data, and are additional parameters, on which our function depends and which have to be learned. When we have this representation , we can use it e.g. to reconstruct the original data (as in unsupervised learning), or to predict some value of interest, (as in supervised learning).
All of , , and are usually numeric arrays, and can be at least stored as vectors and matrices. But storage alone is not important. The important thing is that our function is often linear, that is, . Examples of such linear algorithms are:
- linear regression, where . It is a sensible baseline for regression problems, and a popular tool for answering questions like "does affect , other things being equal?"
- logistic regression, where . It is a good baseline for classification problems, and sometimes this baseline is difficult to beat.
- principal component analysis, where is just a low-dimensional representation of high-dimensional , from which can be restored with high precision. You can think of it as a compression algorithm.
- Other PCA-like algorithms (matrix decompositions) are widely used in recommender systems, to turn a very sparce matrix of "which products were purchased by which users" into compact and dense representations of users and products, that can be further used to predict new transactions.
Other algorithms, like neural network, learn nonlinear transformations, but still rely heavily on linear operations (that is, matrix-matrix or matrix-vector multiplication). A simple neural network may look like — it uses two matrix multiplications, and a nonlinear transformation between them.
Training
To train an algorithm, you usually define a loss function and try to optimize it. The loss itself is sometimes convenient to write in terms of linear algebra. For example, the quadratic loss (used in the least squares method) can be written as a dot product , where is the vector of your prediction, and is the ground truth you try to predict. This representation is useful, because it enables us to derive ways to minimize this loss. For example, if you use linear regression with this least squares method, then your optimal solution looks like . Lots of linear operations in one place!
Another example of linear solution is PCA, where the parameters of interest are the first eigenvectors of the matrix , corresponding to the largest eigenvalues.
If you train neural networks, there is usually no analytical solution for the optimal parameters, and you have to use gradient descent. To do this, you need to differentiate the loss w.r.t. the parameters, and it while doing so, you again have to multiply matrices, because if (a composite function), then , and all these derivatives are matrices or vectors, because and are multidimensional.
Simple gradient descent is OK, but it is slow. You can speed it up, by applying Newtonian optimization methods. The basic method is , where and are are the vector of first derivatives and the matrix of the second derivatives of your loss w.r.t. the parameters . But it can be unstable and/or computationally expensive, and you may need to come up with its approximations (like L-BFGS) that use even more involved linear algebra for quick and cheap optimization.
Analysis
You see that linear algebra helps you to apply and to train your models. But the real science (or magic) starts when your model refuses to train or predict well. The learning may get stuck at a bad point, or suddenly go wild. In deep learning, it often happens due to vanishing or exploding gradients. That is, whey you calculate the gradient, you multiply lots of matrices, and then strange things happen, and you need to know what, why, and how to overcome it. One of the ways to inspect what is happening is to keep track of the eigenvalues of the matrices you are trying to invert. If they are close to 0, or just very different, then the inversion of this matrix can lead to unstable results. If you multiply many matrices with large eigenvalues, the product explodes. When these eigenvalues are small, the result fades to zero.
Different techniques, like L1/L2 regularization, batch normalization, and LSTM were invented in order to fight these problems with convergence. If you want to apply any of those techniques, you need a way to measure whether they help much for your particular problem. And if you want to invent such a technique yourself, you need a way to prove that it can work at all. This again involves lots of manipulation with vectors, matrices, their decompositions, etc.
Conclusion
You can see that the deeper you dive into machine learning, the more linear algebra you see there. To apply pre-trained models, you have to at least convert your data into a format, compatible with linear algebra (e.g. numpy.array
in Python). If you need to implement a training algorithm, or even to invent a new one, be prepared to multiply, invert, and decompose lots of matrices.
In this text, I have referenced some concepts you may be unfamiliar with. It's okay. What this article encourages you is to search for the unknown words and enlarge your horizons.
By the way, it would be interesting to hear some stories from you in the comments, about how you encountered applications of linear algebra in your own job or study.
P.S. In one of my articles, I argued that you don't have to learn maths in order to become successful (it's still a popular stereotype in Russia), even if you work in IT. However, I never said that maths is useless (otherwise, I wouldn't be teaching it all the time). Usually it is not the key to success, but in many cases, it helps, and in a few (like developing deep learning models), it is essential.
P.P.S. Почему на английском?! Ну просто потому что могу. Оригинальный вопрос мне задали на этом языке, и на английском же я на него ответил. А потом решил, что ответ можно довести до уровня маленькой публичной статьюшки.
Почему тогда Хабр, а не, например, Медиум? Во-первых, в отличие от Медиума, тут нормально поддержаны формулы. Во-вторых, Хабр вроде сам собирался выходить на международные рынки — так почему бы не попробовать разместить тут кусочек англоязычного контента?
Посмотрим, что из этого получится.
Sirion
НАЧАЛОСЬ
SilverDY
Хабр хотел начать покорять аудиторию со знанием англ. языка. И круто, что это «НАЧАЛОСЬ» :)
Sirion
на часы, то на еврея, то на часы, то на еврея потом как даст еврею по
морде. С сиденья вскакивает пьяный и тоже начинает бить еврея. Всех
забирают в милицию. Милиционер допрашивает всех по очереди.
Еврей:
— Я ничего не понимаю, вошел в вагон, военный меня ударил,
потом этот пьяный еще набросился.
Милиционер спрашивает у военного:
— За что вы его ударили?
Военный:
— Я спокойно ехал, вошел этот гражданин и встал мне на ногу. Я
подумал, что если он через 5 минут не сойдет с моей ноги, ударю
по морде.
Милиционер спрашивает у пьяного:
— Ну хорошо а вы-то зачем полезли, ведь в другой стороне сидели?
Пьяный:
— Смотрю, сидит военный и смотрит то на часы, то на еврея, то на
часы, то на еврея, потом как даст ему по морде, ну я подумал,
что по всей России началось!
cointegrated Автор
!?? ?????
rkaganov
??????, ??? ?? ?????
scifinder
Граждане, давайте ограничимся только русским и английским! Таки не все вас понимают.
Vinchi
А вот ни фига. Иврит тоже человеческий язык.
codemafia
Почему мне кажется, что вы обсуждаете большой алмаз в какой-то Великобритании?
Yowkis
ПОЧАЛОСЯ
WinPooh73
Nun, mir ist die Sprache egal, gib mir nur gute Inhalte.
MaxVetrov
Warum sprechen Sie Deutsch?
?Hablas espanol?
WinPooh73
Ni ankau povas uzi Esperanton au ec Loglanon.
MaxVetrov
??. Bitte sprechen Sie mir nicht uber Ithkuil =)
Sirion
Если серьёзно, какой-то немного странный вопрос. Как применяется линейная алгебра в {{название области, которая практически состоит из линейной алгебры, как огурец из воды}}?
cointegrated Автор
Это реальный вопрос, который мне задали и залайкали. Я тоже был удивлён. Но коли спрашивают, почему бы не ответить?
3dcryx
I assume that you, the reader, have at least a vague idea of linear algebra concepts (such as vectors, matrices, their products, inverse matrices, eigenvectors and eigenvalues), and machine learning problems (such as regression, classification and dimensionality reduction). If not, maybe now it's a good time to read about them in Wikipedia, or even signup for a MOOC on these subjects.
Серьезно? Тоесть тот кто знаком с основами машинного обучения, линейной ригрессией, задачей классификации и понижением размерности будет задавать вопрос который стоит в загаловке?
pehat
А к Вам ещё ни разу не приходили собеседоваться старшие(!!!) дата-сатанисты, которые не знают, что такое полнота и точность?
firk
По поводу опроса.
По-моему, всё же, если автор русскоязычный и пишет он для русскоязычных, то писать следует на русском. Если же русскоязычный автор пишет на английском, то тут несколько вариантов:
1) он пишет на площадке где аудитория в основном не знает русского языка (это не про хабр)
2) он пишет на русскоязычной площадке но в первую очередь для иностранцев (тут разные обоснования бывают, но в большинстве случаев я бы посчитал это неуважением к основной аудитории — типа, вы тут есть, но я тут ради в первую очередь других)
3) он написал англоязычный дубликат своей русскоязычной статьи
cointegrated Автор
А можно пояснить третью опцию? Она для каких площадок годится?
tvr
Емнип, здесь, на Хабре, несколько раз проскакивали переводы на русский с английского изначально русскоязычных статей.
tvr
В голосовалке понял только один вопрос.
Поэтому пришлось голосовать за третий пункт.
MaxVetrov
I?ve never seen English articles(without translation) on the Habr. Good beginning!
dmagin
Пара замечаний.
1. Функция ошибок, если быть точным, зависит от метрики: Err = (Y-Y')* G (Y-Y'). Тут и далее звездочка обозначает транспонирование.
2. Часто упоминаемое сочетание /(X* X) X* — это ни что иное как псевдообратная_матрица. Здесь левая.
3. Не менее (возможно более) интересна так называемая (data-)resolution matrix, определяемая формулой D = X /(X* X) X*. В терминах статьи данная матрица используется в выражении H = D Y. Чем она «более диагональна», — тем лучше работает линейная модель.
Фишка матрицы в том, что она безразмерна и, как следствие, — не зависит от метрики.
Emulyator
Стоит отметить, что такой текст неплохо переводится и браузерным переводчиком, а «ручные» переводы статей нередко вызывают нарекания в комментариях. Если контент интересный и/или требует обсуждения, то полезно иметь две и более языковых версий, что иногда и так случается благодаря альтруистам-переводчикам. Я «за» англоязычные статьи и против обязательного требования предоставления русской версии, но хотелось бы, чтобы наличие таковой носило рекомендательный характер. Некоторые авторы уже сейчас делают 2 версии (одну в виде ссылки).
Tercel
Me kud spoke england veri bestest.
maisvendoo
Мне кажется посыл смены домена на com и разрешения английского в постах направлен на привлечение в сообщество зарубежных авторов. Но сей посыл не имеет смысла, без наличия на ресурсе англоязычного контента. Сам он тут не появится.
Но! Смысл данной статьи сводится к тому, что линейная алгебра нужна, потому что она много где применяется, и в том числе для машинного обучения. Ну да, линейная алгебра один из столпов Computer Sience и что? Или статья преследует цель исключительно быть статьей на английском, к чему я склоняюсь
cointegrated Автор
На самом деле, целью текста было ответить на конкретный вопрос «what are typical applications of LA in ML» (возникший у не-русскоязычной аудитории). А потом я решил его расшарить публично, и посмотреть, что будет :)
Что аудитория не-русскоязычная — это, наверное, существенно. Всё-таки на постсоветском пространстве существует культ математики, согласно которому математика крайне важна, если не доказано обратное. А вопрошали меня иностранные разработчики, которые про машинку знают больше на уровне
from xgboost import *
или красивых картинок, а теорию никогда особо и не изучали. И если многие наши студенты привычны к формулкам и побаиваются кода, то где-нибудь в Штатах или в Израиле чаще наоборот.roryorangepants
На уровне "import xgboost as xgb" — это "не знают"
Vinchi
По моем не стоит превращать хабр в Quora. А англоязычные статьи здесь есть — просто их переводят, нпример с Medium, так что я за русский.
dimkss
Оф-топик
А как будет выглядеть двуязычный хабр?
Писать и читать его будут только те кто знают оба языка?
cointegrated Автор
Мне кажется, правильное решение — сделать это настраиваемым. Чтобы по умолчанию показывались все статьи, но можно было снимать галочки с языков, на которых читать не хочешь.
Надеюсь, разработчики Хабра так и сделают.
dimkss
Вариант. Но меня смущают ссылки между статьями на разных языках, и, особенно, политика комментирования.
Плюс… допустим понравилась статья. Смотрю в блог автора, а там все статьи на другом языке. Может это и не проблема, но, хоть я и знаю оба языка, но мне было-бы некомфортно.
Zloy_tarakan
А русскоязычная версия будет?
cointegrated Автор
Если этот вопрос апнет куча народу, то можно)
Grinrill
Я не куча народу, но тоже прошу сделать русскоязычную версию)
tangro
Цілком підтримую багатомовність на Хабрі!
gorbin
Имхо — получилось не очень.
Суть даже не в аудитории. просто, как я думаю, сложно читать статью со смешением языков.
То есть вот отличная, может быть, статья, но вокруг так много непонятно текста, ниже обсуждение все на другом языке — вызывает дискомфорт и хочется найти что то более «достоверное», хотя материал может быть полнее и подробнее, но не то.
Если уж делать, то как то и окружение подстраивать под язык статьи.